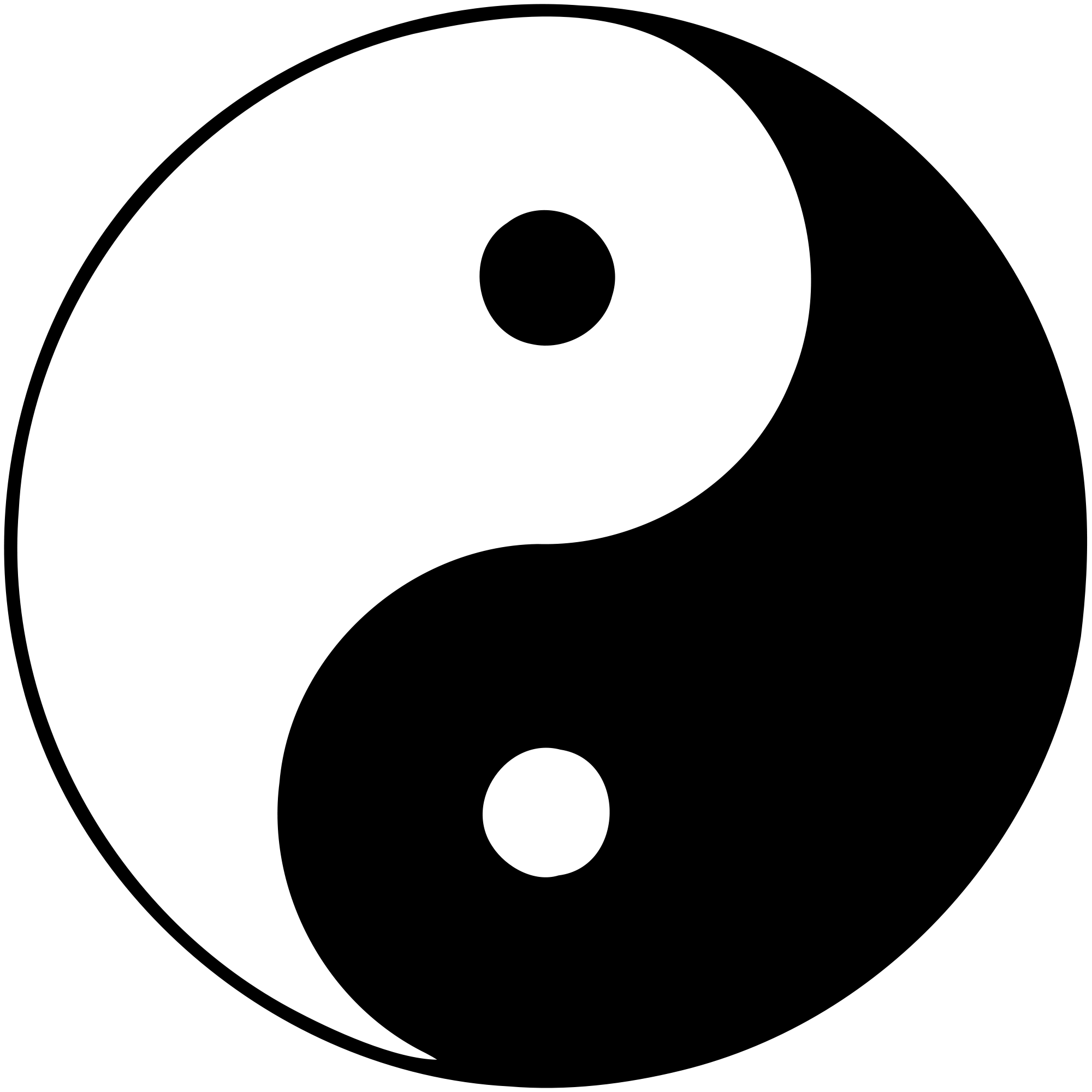
A PhD student has two roles. One is a research assistant that strives to produce good research papers. Another is a learner that keeps improving oneself. Actually, even after obtaining PhD, a researcher still need to learn, and might need to do so for one's lifetime. Research result is explicit while learning result is implicit. That why some students and faculties (e.g. [1]) ignore the later role. However, I would like to use a simple model to show that such ignorance leads to inefficiency.
Let's consider making research progress as a random sampling from a normal distribution, which represents the capability of the PhD student. And higher value of the sample corresponds to higher quality of the research. No matter how many samples are drawn, the average of the samples is the mean of the distribution. And only a few of them could have high value.
Now, student A and B both start with a normal distribution of mean = 1 and variance = 3. And we further assume that sample x > 5 means a very good paper and x < 0 means a failed research project. The figure is:
Now, A decide to solely focus on research. So A keep sampling from this distribution. However, the probability to produce a good pare is only 1.0%. So 100 trials lead to one good paper. And the chance of failure is 28.2%, so more than 1/4 of the trials result in failure.
On the other hand, B focus on learning, by which B pushed the mean of the distribution to 3:
I don't want to draw any conclusion because it is just a very rough model. However, I think you can see the point. And I am also not saying that a PhD student should only focus on learning without any research responsibility. I personally think a PhD student should definitely spend more time on research than on learning. And doing research is actually another very important way of learning, that's why I put the Taiji graph in the beginning of this article, because they boost each other. The point I want to make is that during the journey, sometime there will be a stagnant period during research and we might feel sad. However, we should smile because as long as we keep learning and keep pushing the mean of our normal distribution to the right, things will be fine:)
References:
[1] The picture. http://www.acuherb.us/image/taiji01.png
[2] http://blog.liyiwei.org/?p=1429